My last few posts have been very programming heavy. While I try and get my neural network from my last post up and running, let’s take a break from all that and actually build something.
I’ve previously posted a few projects with pretty complicated code that takes hours to run. One solution was to build a Raspberry Pi cluster which can run code in parallel. But that is far from the fastest way of doing it. I can pretty much hear people screaming at the screen ‘Use the GPU!’, so that’s what we’re going to do here. But first let’s take a step back…
Parallel processing hardware
I’ve posted about exactly what parallel processing is before. In short, it’s breaking a program up into smaller sections and running them all simultaneously. Just writing a program and running it on any normal computer will send it to the CPU. If the program is capable of running in parallel, this is usually the slowest way to run it. With different hardware, it is possible to speed up running times by orders of magnitude. Here are the most common options:

Parallel processing hardware options plotted to show speed vs versatility. The colour of each option is the difficulty to use it (green= easy, red=difficult). The number of dollar signs shows a very rough order of magnitude cost for each option.
Let’s look at each in turn:
Central Processing Unit (CPU) single core
The CPU, or ‘central processing unit’ is the main processor of your computer. It is capable of running basically anything. But it runs programs in sequence, so it has to complete one task before moving onto the next.

How a CPU plots a tetrahedron. For thousands of vertices it’s not difficult to see this is not a feasible method for advanced graphics.
For sequential programs, the CPU is very fast. But we are dealing with parallel programs here.
CPU Multi-Core
Most CPUs today have multiple cores. A core is basically an independent CPU. Unless instructed otherwise, executing a program will send it to just a single core of the CPU. However, if we can run the program in parallel, why not split it up and send it to the separate CPUs separately and run the tasks in parallel? I did this previously with a cluster of 4 Raspberry Pis, each with a quad core CPU, giving a total of 16 cores running in parallel.
Although pretty simple to run, using multiple CPU cores offers a limited speed up potential. For a 4 core CPU, the maximum theoretical possible speed up is 4 times.
General Purpose Graphics Processing Unit Processing (GPGPU)
This is the focus of this post. This approach is essentially an extreme version of multi-core CPU processing.
GPU stands for ‘Graphics Processing Unit’. They became widespread in the 1990’s when PC-based computer gaming became widespread. Prior to GPUs, PCs tended to incorporate relatively fast CPUs, with plenty of memory available, but even a fast CPU was utterly useless for drawing and rendering 3D polygons.
Plotting 3D graphics in real time essentially involves calculating the position for multiple vertices in 3-dimensional space. These vertices are then used as a framework for the subsequent rendering. For a CPU, this is a nightmare. To draw just a single frame the processor must calculate each vertex in turn and only when every vertex has been calculated can it be committed to the screen. CPUs can calculate each vertex quickly but there are thousands in each frame. And we need to plot at least 30 frames per second. Then add the rendering necessary and it’s easy to see how computationally intensive 3D graphics are.
A GPU approaches the problem differently. Rather than a small number of extremely fast cores, as in a CPU, a GPU consists of thousands of slower speed cores. Calculating the position of each vertex takes longer for a GPU core to do compared to a CPU core, but it calculates them all at the same time, so the overall frame is drawn far quicker.

How a GPU plots a tetrahedron. Since the vertices can be plotted simultaneously, the image can be resolved at high speed.
It’s basically the same as asking a maths professor to write down the first 100 multiples of 7, and then asking 100 children, numbered from 1 to 100 to write down their number multiplied by seven. The 100 children would produce all 100 multiples way faster, even though each of them is slower than the professor individually.
But why just use a GPU to draw vertices? Why not use it for…well…whatever you want? That’s what General Purpose GPU programming is about.
This extra speed comes with disadvantages however:
- From a computational standpoint, GPUs are far less flexible than CPUs. They only beat CPUs for programs where a significant portion can be run in parallel. This gives far less flexibility.
- They are difficult to program. Specialist languages (namely CUDA and OpenCL) are necessary for GPU programming. I’ll be posting on these at a later date.
- They produce a lot of heat. Not necessarily an issue but I’ll come back to that in a bit…
Conventionally, the flexibility issue is somewhat mitigated by using a GPU in conjunction with a CPU. The CPU performs the serial sections of the code with the GPU running the parallel sections. This does nothing to reduce the complexity however.
Application Specific Integrated Circuit (ASIC)
At the far end of the speed, complexity and cost scale is the ASIC. An ASIC is just a custom-build chip designed to perform one and only one specific task.
As they must be custom made, and are completely inflexible, the cost is astronomical. Likewise, the design process is complex. A hobbyist would never have an ASIC custom built for one of their own algorithms, however, they are used by hobbyists who wish to perform common tasks.
The classic case of ‘ASICS at home’ is for Bitcoin mining. It’s not something I’ve ever been involved with but it’s an interesting example to illustrate everything we have discussed so far:
- In order to mine Bitcoins, a special algorithm called SHA-256 must be run repeatedly. The faster you can run it, and with the least power usage, the more Bitcoins you mine. This algorithm is suitable for parallelisation.
- Originally early miners used their CPUs to run the algorithm. An ‘arms race’ started. Those who could mine the fastest took almost all the profits.
- Miners moved onto using their GPUs, as discussed above.
- Then it was realised specially designed circuits could mine Bitcoins far faster than GPUs. These are ASIC Unlike an ASIC with only one application and one user, it is profitable to design and sell them because there are many people worldwide who mine Bitcoin.
It must be emphasised that once your Bitcoin mining ASIC is obsolete it is scrap. It’s not like a year old (they go obsolete in a matter of months) GPU which can be used for something else. They can just do one thing.
So ASICs are sometimes used by amateurs but certainly not designed by them right? Not exactly:
Field Programmable Gate Arrays (FPGAs)
FPGAs are a sort of ‘blank canvas’ on a chip. Using a special language, they can be programmed to re-arrange their internal components to emulate any device which can be built from that number of components. Want to build a CPU from scratch? You can do it with an FPGA. An ASIC to run a specific algorithm? Absolutely possible.
Although more expensive than a typical GPU, FPGAs can basically take the role of any of the other methods described here.
The catch is obvious though: they are horrendously difficult to use. It doesn’t take a giant leap of imagination to realise that designing a circuit, then programming it into the FPGA, then programming your algorithm into that is going to involve a lot of work.
Nevertheless, FPGAs are certainly becoming accessible to hobbyists. I doubt I’ll be learning to use them in the immediate future, but when I do, I’ll be posting about it here!
Building My System
That’s enough background. Let’s look at what I have been up to.
My problem is that I want to learn GPU programming, and use it to speed up computations. In order to do that I need a reasonably powerful GPU. However, to run a powerful GPU, I’d need a decent desktop computer. To run anything half decent, I’d be looking at a $1500 system. This is clearly a waste of money, is there a way to connect a decent GPU up to my cheap laptop?
My laptop
It’s worth noting that my laptop is absolutely not designed for this kind of thing. It just about manages to run Kerbal Space Program OK. For those interested, it has an AMD R5 GPUand A8 CPU.
I removed the CD drive from it a few months ago because who uses CD drives anymore? I was going to use it for a second hard drive but I don’t really need that. The space will come in handy here.
Surely this won’t work…
A few weeks ago I came across this video from DIY Perks:
In essence, it’s a device which plugs into a laptop’s PCI-E port. The PCI-E port is where the Wifi card is normally plugged in. Swapping out the Wifi card for this device allows you to plug in a graphics card and run it externally from the laptop.
I was highly skeptical about this. I mean, there doesn’t seem to be a reason for it to not work, but it just doesn’t feel like it should. Anyway, I ordered one from a seller in Thailand. It’s not the same as the one in the video (they were sold out) but exactly the same type of thing.
The GPU that I want to connect to the laptop
I got myself on Ebay to find a good GPU. I didn’t want something top of the range, but something decent and up to date. In the end I went with an AMD A9 290. Feel free to look up the specs yourself, you’ll probably be able to make more sense of them that I can.
However…
The one I actually bought has a bit more going for it. I still don’t know why, but there was one with an integrated water cooling system going for cheap. Being an engineer, I had to get that one!

My new graphics card with water cooling system. It is normally connected to a desktop motherboard via the pins on the bottom (most aren’t visible here)
Earlier I mentioned that GPUs kick out an awful lot of heat. Folk who are seriously into their gaming often try and run their GPUs as fast as possible (ie overclocking). Using just fans for cooling under these circumstances is noisy at best, and ineffective at worst. So, the solution is to water cool the GPU.
I cannot stress how unnecessary this is. I only bought it because it is cool. I barely ever play computer games and this system isn’t going to be used for gaming (much). But I suppose it’ll let me overclock it if I want to speed up my programs even more.
Putting it together
Here is the GPU connected to the adapter. The GPU needs a fair amount of power so I’ve connected it to a standard PC power supply. Highlighted also are the power connectors for the water pump and fans – I just plugged them into the MOLEX (ie 12v power) connectors via an adapter I got hold of for less than $3 on Ebay.
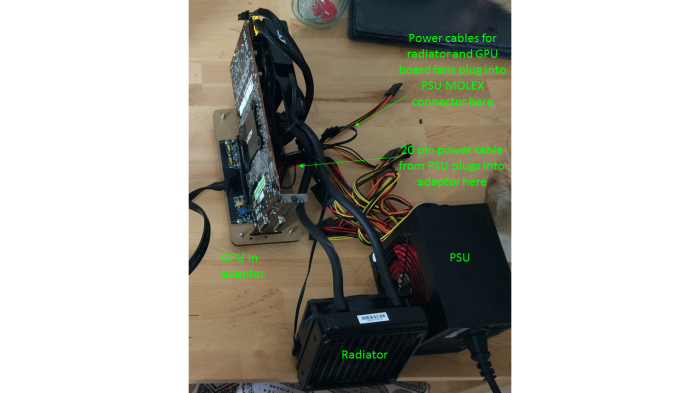
It looks like a mess but it’s cheap and I can worry about putting it in a case later
Now I need to plug the PCI-E connection from the adapter into my laptop somehow.
Right, let’s open it up!
There aren’t many things I own that I haven’t taken to pieces so this was all pretty simple. Here’s the inside of the laptop with the WIFI card labelled. I needed to somehow make this location of the motherboard accessible so I could swap between the Wifi card and the GPU adapter:

Inside the laptop. A graphics card can be plugged into the labelled slot with the adapter.
The obvious thing to do was to run a PCI-E extension from the current location to the empty CD drive bay. This would make the port accessible so I can change between the Wifi card and the GPU adapter.

In order to make the graphics card and wifi card interchangeable I decided I’d need to run an extension cable from the existing slot to the now empty CD drive bay, as shown here.

Here is the PCI-E cable I used. Unfortunately I forgot to take a photo of it in place.
There was one issue. The Wifi card has an antenna attached. The antenna cable originally ran up the inside of the monitor and connected to the antenna. It is not long enough to reach from the new position of the Wifi card to the existing Wifi antenna.
Since the Wifi card is now going to be positioned in the CD drive bay, I just removed the antenna and re-positioned it behind the right hand side of the monitor. The cable now runs down the other side of the screen and into the laptop body through a little hole I cut into the hinge.

With the screen removed, we can see the Wifi antenna behind it. Highlighted is the position I moved it to in order to allow re-routing of the antenna cable. The antenna was glued in place so I just peeled it off and stuck it in the new position.

How the antenna was re-positioned behind the screen. Bonus nerd points for identifying what my wallpaper is!
I attached the extension to the inside of the laptop body and put everything back together. After installing a removable cover (originally the front of the CD drive), here’s what we have:

The new GPU connected to the laptop and ready to go. It can be readily swapped out for the Wifi card (shown next to the plug in point).
I put it all into an enclosure to stop it being a total mess, and that’s it done!

In a case with the covers removed. I could have used a far smaller enclosure but this one was extremely cheap and does the job just fine.
Does it work?
So, let’s swap out the wifi card for the GPU adaptor, plug in a monitor and turn everything on:
After downloading the drivers and connecting the GPU to a monitor, here’s what showed up in my graphics card settings:

The new GPU is detected by the system, so things are looking good! Note the standard R5 GPU has been relegated to ‘secondary GPU’.
Well, the GPU is detected so that’s a good start. But does it actually work? Let’s write some code to find out…
…Nah, let’s play Doom!

Of course I did this to learn more about GPGPU processing. Doom had nothing to do with this project. Nope, nothing at all…
Amazingly, this ridiculous setup actually works flawlessly. I’m not much into gaming but I do make an exception for Doom, it was basically my childhood. One of my proudest moments was getting told off for installing the original game on the computers at my junior school when I was 9. This was in the days that people thought ‘violent computer games’ would turn their kids into Patrick Bateman.
Anyway, it runs perfectly, without the new graphics card the game didn’t even start up.
I’ll be revisiting this is the relatively near future in order to write some programs that actually run on the GPU. In order to do so I need to learn OpenCL which looks like it will take a bit of time.