I was wrong; but not by much…
Updates
09/11/2016 – 19:30 GMT. As discussed earlier, I’ll be doing this again in the future. I’ve updated the main page with the reasons why I was wrong and how I will improve on it next time. I’ll do a final update in a few days when the final count is in.
09/11/2016 – 12:00 GMT. Well I was wrong. As stated when I made my prediction, this does not surprise me at all. While most poll aggregators were putting Hillary’s chance of winning at around 70%, I put it at just 52.5%. Clearly, my model is not acceptable – I still made the wrong prediction, and at a very low confidence level too; but it was ‘less wrong’ than the polls! The day prior to the election, my model’s prediction almost switched back to Trump – I’m a bit gutted that it didn’t!
I’m still very much interested in the final vote count. My prediction of Hillary taking 52.5% of the non-third party votes still stands so let’s see how I do there.
As I keep stating, the accuracy of my model was way below an acceptable level for good predictions (but it was still higher than the polls!). This is largely due to the limited amount of data I used – the wordcount from a single newspaper and 10 different stocks over 6 months.
Most importantly for me, this has been a proof of concept. So I will definitely be doing this again for the next major worldwide election or referendum. Only next time I will be using at least 10,000 times the amount of data to predict on, and I will be trialling hundreds of models
More updates on how wrong I was and how I will fix it in the future to follow.
08/11/2016 22:30 GMT. Here is today’s wordcount (in red) for the top 125 words, as used in the model from the New York Times, and the average wordcount over the past 6 months (in black). Maybe I was a bit hasty to dismiss todays prediction on the grounds that the wordcount would be exceptional today – it looks pretty close to normal.
That said, there are a few huge outliers. Maybe these threw my model’s prediction this morning, or maybe not…
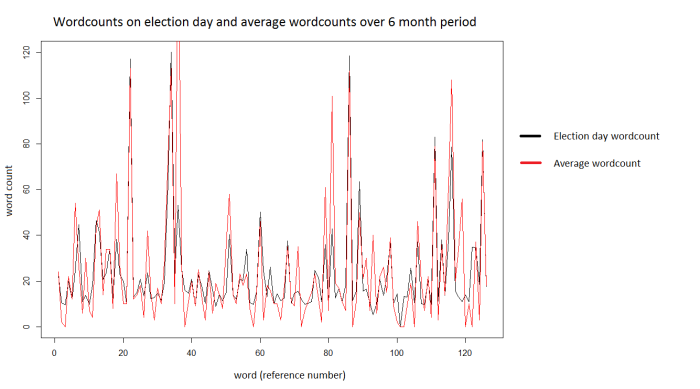
Each of the 125 words used as an input for the model occupies a ‘slot’ on the x axis. A bar plot would be clearer but would have had 125 bars so would have been a nightmare to read. Unsurprisingly: the most used word today was ‘election’ (the huge red peak)
As discussed, today’s input data predicted a Hillary win more strongly (>60%) than the prediction I committed to. So, the stock market data I just posted is at odds with my model. As discussed below, I’m not reading anything into this.
08/11/2016 21:40 GMT. The stock market has closed, since I am using it as my proxy for polling data, the results are relevant to us. Looking at the stocks I chose to form indices which favoured a Republican or Democrat victory, here are the % changes they closed on:
Democrat | Republican | |||
% change | % change | |||
SCTY | 1.56 | XOM | 0.72 | |
NOC | -0.08 | SWHC | 2.15 | |
INTC | 0.14 | BHP | 3.23 | |
AVP | -0.01 | GEO | -0.08 | |
BA | 0.18 | GOLD | -0.24 | |
TOTAL | 1.79 | TOTAL | 5.78 |
Taken alone this would predict Trump to win (based on the weak assumption that these shares are representative). However, the stock data on it’s own is a very weak indicator – this is why we built a prediction model in the first place. This is also a single data point taken on a very abnormal day. Generally all stocks rise on average if the party currently in power is predicted to win. And, investors generally currently favour Hillary. So really there is very little we can take from this.
Still, it’s something to keep everyone on their toes – Hillary is far from safe yet!
My model
A more comprehensive description of the model is given in the previous post. It used a fast Adaboost algorithm to predict the performance of chosen US stocks. The input data was the counts of the 125 most common words on the front of the New York times. The assumption being that certain stocks perform better depending on who the market believes will win the election. In theory, the contents of the daily news should be able to predict that.
Clearly, the above is subject to high levels of noise, hence the use of an ensemble learning method. Other methods were tried (support vector machine, boosted C5.0 tree) but Adaboost consistently gave a higher model accuracy on the test data.
The key point is that this model did not rely on poll data which is presumed to be unreliable.
My prediction
As stated in the previous post, my final prediction was Hillary to win, with 52.5% of the non-third party candidate votes. The model accuracy is low, at just 63.6%, but it’s better than guessing.
Here’s a look at what the model predicted in the couple of days before the election:

The predictions I made prior to the election. I went with the second to last one as my final estimate of the of the share
The main points of interest here are:
- Trump was initially predicted to win, but the FBI announced no criminal action by Hillary in relation to her emails fiasco. This put Hillary ahead right away. She made a significant gain the next day as the New York Times published a front page article on this.
- The model accuracy was drastically improved to 63.6% with the second to last estimate. This was by changing the variation of the adaboost algorithm used and, probably more importantly, weighting each training data instance on the data from the previous 5 days. In other words, rather than just looking at that day’s news, previous days were also considered.
- Hillary had a large jump in probability of winning on the day of the polls. I chose not to use this for my final prediction as the New York Times data was probably less comparable to that of previous days.
- The main aspect to stress is that the model accuracy is still very low. I hope to improve this model in the future to predict other elections but it will need significant improvement – at present it uses just one newspaper and an index of 5 stocks for each candidate. Ideally in future, that 1 newspaper will be replaced with every (yes, every) tweet posted that day, or something like that.
Why was I wrong?
The main reason was simply a lack of data. The model was thrown together in a day and only drew data from 10 US stocks and the front page of the New York Times. This was not enough to build a model with a significant level of confidence.
I did acknowledge this ahead of the election and am not at all surprised my prediction was wrong. Importantly for me, this was a good proof of concept. If I can throw that together in a day, I should be able to do far cooler things in the future!
But, I wan’t that wrong
My consolation is that I only predicted a Hillary win with 52.5% confidence. The day before the election, most polls were putting her probability of winning at around 70%. So, I did call it being a lot closer than the polls did.
Limitations
Unfortunately, I couldn’t do anything cool like try and call states ahead of news agencies. I’ve simply not had the time to build a more complex model. I’ll try for that at the next election though.
I couldn’t predict who will win the Senate or House of Representatives either – I’m just predicting the popular vote here. In addition, I’m only predicting the relative votes for Hillary and Trump and am no predicting third party candidates.
Also, the model used is a classifier rather than a regression model. That means it is not designed for numerical estimates. That is why I stress the vote count estimate is more of an educated guess.
Some other things I’ve learned from this
- There are some very weird rules regarding voting. I was astonished to find out that some states allow voters to take photos of their ballot paper which basically opens up the door for vote selling.
- Likewise, there is no restriction on publishing exit poll data whilst the vote is ongoing. That is strictly forbidden in the UK as it may influence the vote.
- I also find it concerning that votes are taken electronically. Tom Scott has an excellent video regarding how exploitable this is. Maybe Trump has a point after all about things being rigged – shame he just decides to shout about it rather than provide any form of actual argument.
- Both Randall from xkcd and Henry from MinutePhysics are among normally non-political folk who have publicly endorsed Hillary . This is really unusual; personally I have no issue with it, especially given the circumstances of this particular election, but it does go to show how unusual the past few months have been.
None of the above is me deriding America by the way, just some observations which really surprised me.
What next?
The real take away point from this (which I think we all knew before) is that predicting the election with no polling data requires either ingenuity, or an enormous amount of data. I am used to dealing with the latter.
I’ve already had some really positive feedback on the previous post and really enjoyed getting involved. I’ll certainly be using a similar (vastly improved) model for more predictions in the future. Except, rather than building a model on a few thousand words per day, I’ll be using millions. And instead of using 10 stocks as poll proxies, I’ll be using thousands. I’ll probably need multiple GPUs on Amazon EC2 (or equivalent) to train the model but I can do that.

The relative sizes (by area) of the training/testing dataset I used for this election and the size of a large sample of tweets posted in one day – the sort of thing I plan to use to build a model next time. The size difference is around 4 orders of magnitude
This model was a prototype, the next one will be huge in comparison.
Pingback: US election prediction with boosted decision trees | brain -> blueprint -> build